#364: Grimes Embraces AI, But Copyright Challenges Loom, & More
1. Grimes Embraces AI, But Copyright Challenges Loom
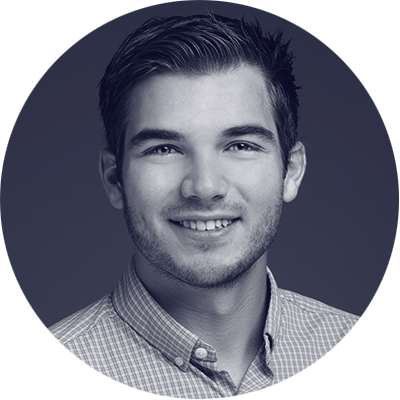
Pop singer Grimes has invited musicians to create new songs by cloning her voice using artificial intelligence (AI) and to split royalties 50-50 with her. While the music industry is focusing on copyright issues associated with new AI-generated songs trained on artists’ voices, Grimes is independent and seemingly happy to be a first mover in commercializing the genre.
The music labels are taking a close look at another AI-generated song featuring Drake and The Weeknd. Although copyright law associated with AI-generated art is murky, Universal Music recently petitioned streaming services to remove that song, arguing that training generative AI with their artists’ voices violates copyright law.
While musicians like Grimes, Holly Herndon, and YACHT have embraced AI as a creative tool, AI-generated music is causing questions around consent, ethics, and creative labor. Creatives experimenting with AI could contribute meaningfully to their resolution and to the evolution of entertainment.
2. Tesla Is Investing More In Electric Vehicles Than Most Traditional Automakers
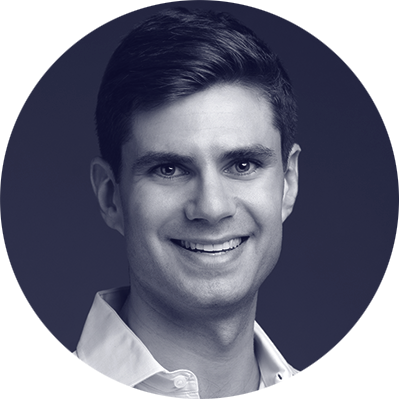
For years, Tesla critics have posited that traditional automakers will ramp electric vehicle (EV) investments so aggressively that Tesla will lose its competitive position. What they did not understand is that traditional automakers would not take EVs seriously soon enough and that Tesla would outspend them at scale. In its 10-Q filing last Monday, Tesla revealed that it had increased its capital expenditure (capex) forecast for 2023 from $6-8 billion to $7-9 billion, a range above which only Ford and Volkswagen—biased up by including research and development—have invested in absolute terms on average per year during the past three years, as shown here.1
Importantly, capex is an absolute dollar amount, not a measure of capital efficiency. ARK’s research suggests that last year Tesla invested ~$7,000 per incremental unit of capacity, roughly half the industry average. In our view, in 2027, its capital efficiency will improve to ~$2,000, putting Tesla not only near the top of the auto capex league table but also at the top of the auto capital efficiency league table.
[1] For informational purposes only and should not be considered investment advice or a recommendation to buy, sell, or hold any particular security or cryptocurrency. Past performance is not indicative of future results.
3. Targeting Intrinsically Disordered Proteins (IDPs) Could Broaden The Druggable Proteome
Deep learning models like AlphaFold 2 (AF2) have revolutionized scientists’ ability to predict the 3D structures of proteins from their primary amino acid sequences. Imperfect, however, AF2 struggles to predict1 the intrinsically disordered regions (IDRs) in ~60% of human proteins.2 AF2 yields confident structural predictions for only 15% of IDRs.3
IDRs are ubiquitous and could be good drug targets. Many diseases arise from mistakes that occur in cell signaling pathways. Indeed, the majority of FDA-approved4 small-molecule and biologic drugs target proteins involved in cell signaling. Researchers estimate that ~70% of human proteins involved in cell signaling contain IDRs.5 Better methods for characterizing IDRs could broaden the number of druggable proteins.
Earlier this month, scientists at the University of Washington’s Institute for Protein Design published an improved method for targeting IDRs. With a physics-based approach, the team aligned the backbones of proteins geometrically and found the most stable match between a target protein and a designed protein-based drug. While based on proteins with repetitive amino acid sequences (e.g., …PLPPLPPLP…), the researchers showed experimentally that the method can generalize to IDRs containing just a few repeats. ARK believes this approach will pave the way for more research into sequence-based targeting of IDRs, potentially catalyzing drug discovery for more disease-associated proteins.
[1] Alderson, T.R. et al. 2022. Systematic identification of conditionally folded intrinsically disordered regions by AlphaFold2. bioRxiv. Available here.
[2] Saurabh, S. et al. 2023. “Fuzzy Drug Targets: Disordered Proteins in the Drug-Discovery Realm.” ACS Omega. Available here.
[3] Alderson, T.R. et al. 2022. Systematic identification of conditionally folded intrinsically disordered regions by AlphaFold2. bioRxiv. Available here.
[4] The Human Protein Atlas. “The Druggable Proteome.” Available here.
[5] Saurabh, S. et al. 2023. “Fuzzy Drug Targets: Disordered Proteins in the Drug-Discovery Realm.” ACS Omega. Available here.