#337: ARK Extends An Open Letter To The Fed, & More
1. ARK Extends An Open Letter To The Fed
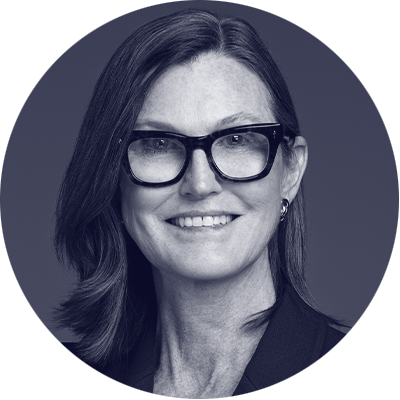
In ARK’s latest In The Know video, out of concern that the Fed is making a policy error that will cause deflation, we offered some data for our “data-driven” Fed to consider as it prepares for its next decision on November 2. In the face of conflicting data, the unanimity of the Fed’s last decision to increase the Fed funds rate by 75 basis points was surprising.
In our latest market commentary article, we delineate first the upstream price deflation that is likely to turn into downstream deflation. Then, we focus on the two variables––employment and headline inflation––upon which the Fed seems to be making its decisions. In our view, both are lagging indicators. Please read the full article here.
2. Tesla Demonstrated Its AI Leadership During AI Day Last Week
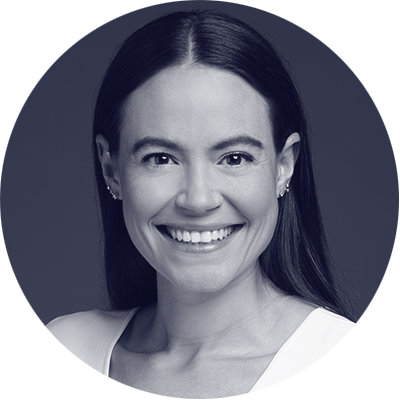
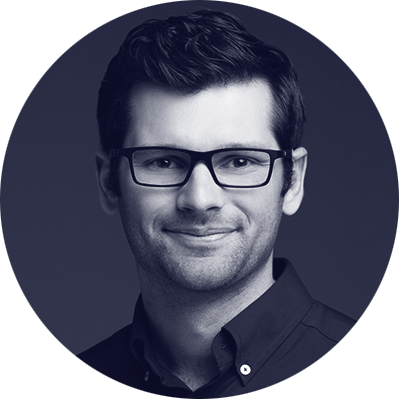
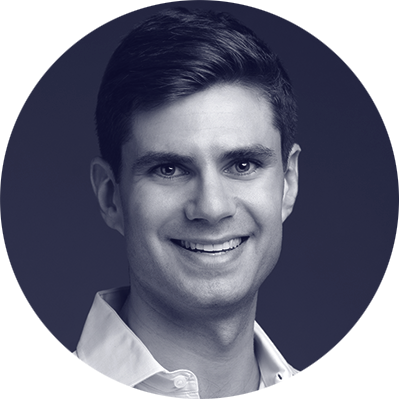
Tesla’s recent AI day highlighted its focus on the pace of innovation. In the coming years, the company hopes to deliver a $20,000 production version of its Optimus robot. To do so, Tesla has reconfigured and retrained Autopilot’s hardware and neural networks. The humanoid robot will operate in environments much less constrained than the roads on which cars drive, presenting a different set of challenges for engineers to solve.
AI hardware? Autonomous vehicles? Humanoid robots? What company could be more interesting and challenging than Tesla for innovators who want to move fast, work hard, and deploy at scale.
During AI Day, Elon Musk re-committed to the worldwide rollout of Tesla’s Full Self Driving (FSD) software by the end of this year. Tesla continues to push more of its autonomous driving software into neural nets that are governed by deep learning instead of manually created rules. The company also revealed that other AI models, including language models, have inspired its methods to create digital representations of roads and intersections. With roughly three million cars providing massive reservoirs of global training data, Tesla is likely to be one of the few companies capable of developing a foundation model for objects that move through physical space.
Tesla also disclosed significant progress on its Dojo supercomputer. Its existing supercomputer, powered by Nvidia’s A100 accelerators, takes more than a month to train Tesla’s largest neural networks. Dojo reduces the training time to less than a week, giving engineers much more time to iterate key models like auto-labelling and the occupancy network.
To achieve those performance gains, Tesla designed Dojo with the compute density and bandwidth necessary to train large neural networks. Leveraging high-bandwidth interconnects and minimizing the physical distance between chips, Tesla reduces latency and organizes many chips to function as a single unit of compute. One Dojo “tile” combines twenty-five chips to deliver the performance of up to six GPU-based servers––all for less than the cost to buy one server. Achieving that level of density has come with challenges that require custom power, cooling, and packaging solutions. With those compiler optimizations, our research suggests that Dojo should be 3.2x to 4.4x more powerful on a per-chip basis than Nvidia’s existing training hardware. Next quarter, Musk hopes to deliver Dojo’s first production use case and, with its next generation of hardware, is planning for even greater acceleration.
3. Moving-Image Modeling Is Breaking Out With Make-A-Video, Imagen Video, Phenaki, DreamFusion, And Stable-DreamFusion
Artificial intelligence breakthroughs in the moving-image space have exploded in recent months, compounding as researchers and developers in one area apply and feed rapid success in others. The research is sizzling: according to Mario Krenn, the number of AI-related papers on arXiv is growing exponentially.
In last week’s ARK Newsletter, we discussed Meta Platforms’ Make-A-Video text-to-video model. Three days later, Google Research published research on its new text-to-video model, Imagen Video. Here we analyze their differences.1
Meta constructed Make-A-Video using not only a basic text-to-image model trained on text-image pairs but also unsupervised learning on unpaired video footage. Google took a slightly different approach, using its proprietary Imagen text-to-image model, trained on text-image pairs, in conjunction with the publicly available LAION-400M image-text dataset and a proprietary dataset of 14 million video-text pairs. At first glance, their output looks similar. Our analysis suggests that Google’s Imagen performs better at delivering videos that move through 3D space holistically, while Meta’s Make-A-Video’s videos contain isolated movement in otherwise static images. Imagen Video also seems to deliver English text in a highly coherent manner, especially compared to the DALL-E 2 and Stable Diffusion text-to-image models––both of which struggle when rendering text.
Alongside Imagen Video, Google Research also published research on Phenaki, its new text-to-video model that generates longer videos using a sequence of user-generated text prompts. Although the model sacrifices visual fidelity for length, Phenaki appears to generate good-enough videos lasting a few minutes and depicting time sequences more effectively than both Make-A-Video and Imagen Video. Phenaki’s challenge is to deliver higher-resolution outputs while minimizing computational costs. We are looking forward to exploring the possible synergies between Imagen Video with Phenaki.
The models enabling Make-A-Video, Imagen Video, and Phenaki represent an explosion in AI innovation. We will continue to monitor the commercialization potential of text-to-video models, including their ability to create video content that delights consumers.
Meanwhile, In 3-D Land…
Google Research and UC Berkeley published research on their new text-to-3D model, DreamFusion, the same day Meta published Make-A-Video. DreamFusion’s text-to-3D uses Imagen as a pretrained text-to-image model in conjunction with another 3D model called a Neural Radiance Field, or NeRF. The model generates 2D images from different angles, enabling the construction of 3D assets that computer graphics software like Blender or Unity can support. Like Make-A-Video, DreamFusion doesn’t require a large training set of paired data that matches model output: Make-A-Video was not trained on a dataset of video-text pairs, and DreamFusion was not trained on a dataset of 3D asset-text pairs.
In our view, text-to-3D models could have a profound impact on video game development, user-generated content, and in-game advertising. Based on our preliminary estimates, global gaming software and services revenues could approach $200 billion as video game development costs top $100 billion in 2022.
The commercialization of text-to-3D models, however, is likely to disrupt our forecasts: AI models could lower the costs of video game design and development dramatically while the adoption of user generated content (UGC) lowers the barriers to entry. More consumer brands are likely to experiment with immersive in-game advertising, like those advertising on Roblox during the past few years. Moreover, digital ad dollars are likely to move into video games at an accelerated rate if text-to-3D models evolve toward the production of full virtual environments that can host cost-efficient, immersive, programmatic in-game advertising.
Healthy competition among open-source and private models has been key to the rapid advancements in AI recently. One week after the publication of DreamFusion, individual contributors replicated and open-sourced the text-to-3D model using Stable Diffusion, the publicly available text-to-image model replacing Google’s proprietary Imagen model. Now, Stable-Dreamfusion is a work-in-progress toward matching the output quality of DreamFusion. We are marveling at the AI advancements of these past few weeks.
[1] Because Meta Platforms and Google Research haven’t yet released their models for public use, we compare them based on the examples displayed on their respective websites.